Wednesday, December 16, 2009
Monday, December 14, 2009
Friday, December 11, 2009
Kernel Korner - Sleeping in the Kernel
July 28th, 2005 by Kedar Sovani in
* Linux Journal
The old sleep_on() function won't work reliably in an age of SMP systems and hyperthreaded processors. Here's how to make a process sleep in a safe, cross-platform way.
Average:
Cancel rating
Poor
Okay
Good
Great
Awesome
Your rating: None Average: 4.5 (30 votes)
In Linux kernel programming, there are numerous occasions when processes wait until something occurs or when sleeping processes need to be woken up to get some work done. There are different ways to achieve these things.
All of the discussion in this article refers to kernel mode execution. A reference to a process means execution in kernel space in the context of that process.
Some kernel code examples have been reformatted to fit this print format. Line numbers refer to lines in the original file.
The schedule() Function
In Linux, the ready-to-run processes are maintained on a run queue. A ready-to-run process has the state TASK_RUNNING. Once the timeslice of a running process is over, the Linux scheduler picks up another appropriate process from the run queue and allocates CPU power to that process.
A process also voluntarily can relinquish the CPU. The schedule() function could be used by a process to indicate voluntarily to the scheduler that it can schedule some other process on the processor.
Once the process is scheduled back again, execution begins from the point where the process had stopped—that is, execution begins from the call to the schedule() function.
At times, processes want to wait until a certain event occurs, such as a device to initialise, I/O to complete or a timer to expire. In such a case, the process is said to sleep on that event. A process can go to sleep using the schedule() function. The following code puts the executing process to sleep:
sleeping_task = current;
set_current_state(TASK_INTERRUPTIBLE);
schedule();
func1();
/* The rest of the code */
Now, let's take a look at what is happening in there. In the first statement, we store a reference to this process' task structure. current, which really is a macro, gives a pointer to the executing process' task_structure. set_current_state changes the state of the currently executing process from TASK_RUNNING to TASK_INTERRUPTIBLE. In this case, as mentioned above, the schedule() function simply should schedule another process. But that happens only if the state of the task is TASK_RUNNING. When the schedule() function is called with the state as TASK_INTERRUPTIBLE or TASK_UNINTERRUPTIBLE, an additional step is performed: the currently executing process is moved off the run queue before another process is scheduled. The effect of this is the executing process goes to sleep, as it no longer is on the run queue. Hence, it never is scheduled by the scheduler. And, that is how a process can sleep.
Now let's wake it up. Given a reference to a task structure, the process could be woken up by calling:
wake_up_process(sleeping_task);
As you might have guessed, this sets the task state to TASK_RUNNING and puts the task back on the run queue. Of course, the process runs only when the scheduler looks at it the next time around.
So now you know the simplest way of sleeping and waking in the kernel.
Interruptible and Uninterruptible Sleep
A process can sleep in two different modes, interruptible and uninterruptible. In an interruptible sleep, the process could be woken up for processing of signals. In an uninterruptible sleep, the process could not be woken up other than by issuing an explicit wake_up. Interruptible sleep is the preferred way of sleeping, unless there is a situation in which signals cannot be handled at all, such as device I/O.
Lost Wake-Up Problem
Almost always, processes go to sleep after checking some condition. The lost wake-up problem arises out of a race condition that occurs while a process goes to conditional sleep. It is a classic problem in operating systems.
Consider two processes, A and B. Process A is processing from a list, consumer, while the process B is adding to this list, producer. When the list is empty, process A sleeps. Process B wakes A up when it appends anything to the list. The code looks like this:
Process A:
1 spin_lock(&list_lock);
2 if(list_empty(&list_head)) {
3 spin_unlock(&list_lock);
4 set_current_state(TASK_INTERRUPTIBLE);
5 schedule();
6 spin_lock(&list_lock);
7 }
8
9 /* Rest of the code ... */
10 spin_unlock(&list_lock);
Process B:
100 spin_lock(&list_lock);
101 list_add_tail(&list_head, new_node);
102 spin_unlock(&list_lock);
103 wake_up_process(processa_task);
There is one problem with this situation. It may happen that after process A executes line 3 but before it executes line 4, process B is scheduled on another processor. In this timeslice, process B executes all its instructions, 100 through 103. Thus, it performs a wake-up on process A, which has not yet gone to sleep. Now, process A, wrongly assuming that it safely has performed the check for list_empty, sets the state to TASK_INTERRUPTIBLE and goes to sleep.
Thus, a wake up from process B is lost. This is known as the lost wake-up problem. Process A sleeps, even though there are nodes available on the list.
This problem could be avoided by restructuring the code for process A in the following manner:
Process A:
1 set_current_state(TASK_INTERRUPTIBLE);
2 spin_lock(&list_lock);
3 if(list_empty(&list_head)) {
4 spin_unlock(&list_lock);
5 schedule();
6 spin_lock(&list_lock);
7 }
8 set_current_state(TASK_RUNNING);
9
10 /* Rest of the code ... */
11 spin_unlock(&list_lock);
This code avoids the lost wake-up problem. How? We have changed our current state to TASK_INTERRUPTIBLE, before we test the condition. So, what has changed? The change is that whenever a wake_up_process is called for a process whose state is TASK_INTERRUPTIBLE or TASK_UNINTERRUPTIBLE, and the process has not yet called schedule(), the state of the process is changed back to TASK_RUNNING.
Thus, in the above example, even if a wake-up is delivered by process B at any point after the check for list_empty is made, the state of A automatically is changed to TASK_RUNNING. Hence, the call to schedule() does not put process A to sleep; it merely schedules it out for a while, as discussed earlier. Thus, the wake-up no longer is lost.
Here is a code snippet of a real-life example from the Linux kernel (linux-2.6.11/kernel/sched.c: 4254):
4253 /* Wait for kthread_stop */
4254 set_current_state(TASK_INTERRUPTIBLE);
4255 while (!kthread_should_stop()) {
4256 schedule();
4257 set_current_state(TASK_INTERRUPTIBLE);
4258 }
4259 __set_current_state(TASK_RUNNING);
4260 return 0;
This code belongs to the migration_thread. The thread cannot exit until the kthread_should_stop() function returns 1. The thread sleeps while waiting for the function to return 0.
As can be seen from the code, the check for the kthread_should_stop condition is made only after the state is TASK_INTERRUPTIBLE. Hence, the wake-up received after the condition check but before the call to schedule() function is not lost.
Wait Queues
Wait queues are a higher-level mechanism used to put processes to sleep and wake them up. In most instances, you use wait queues. They are needed when more than one process wants to sleep on the occurrence of one or more than one event.
A wait queue for an event is a list of nodes. Each node points to a process waiting for that event. An individual node in this list is called a wait queue entry. Processes that want to sleep while the event occurs add themselves to this list before going to sleep. On the occurrence of the event, one or more processes on the list are woken up. Upon waking up, the processes remove themselves from the list.
A wait queue could be defined and initialised in the following manner:
wait_queue_head_t my_event;
init_waitqueue_head(&my_event);
The same effect could be achieved by using this macro:
DECLARE_WAIT_QUEUE_HEAD(my_event);
Any process that wants to wait on my_event could use either of the following options:
1.
wait_event(&my_event, (event_present == 1) );
2.
wait_event_interruptible(&my_event, (event_present == 1) );
The interruptible version 2 of the options above puts the process to an interruptible sleep, whereas the other (option 1) puts the process into an uninterruptible sleep.
In most instances, a process goes to sleep only after checking some condition for the availability of the resource. To facilitate that, both these functions take an expression as the second argument. The process goes to sleep only if the expression evaluates to false. Care is taken to avoid the lost wake-up problem.
Old kernel versions used the functions sleep_on() and interruptible_sleep_on(), but those two functions can introduce bad race conditions and should not be used.
Let's now take a look at some of the calls for waking up process sleeping on a wait queue:
1.
wake_up(&my_event);: wakes up only one process from the wait queue.
2.
wake_up_all(&my_event);: wakes up all the processes on the wait queue.
3.
wake_up_interruptible(&my_event);: wakes up only one process from the wait queue that is in interruptible sleep.
Wait Queues: Putting It Together
Let us look at a real-life example of how wait queues are used. smbiod is the I/O thread that performs I/O operations for the SMB filesystem. Here is a code snippet for the smbiod thread (linux-2.6.11/fs/smbfs/smbiod.c: 291):
291 static int smbiod(void *unused)
292 {
293 daemonize('smbiod');
294
295 allow_signal(SIGKILL);
296
297 VERBOSE('SMB Kernel thread starting '
'(%d)...\n', current->pid);
298
299 for (;;) {
300 struct smb_sb_info *server;
301 struct list_head *pos, *n;
302
303 /* FIXME: Use poll? */
304 wait_event_interruptible(smbiod_wait,
305 test_bit(SMBIOD_DATA_READY,
&smbiod_flags));
...
... /* Some processing */
312
313 clear_bit(SMBIOD_DATA_READY,
&smbiod_flags);
314
... /* Code to perform the requested I/O */
...
...
337 }
338
339 VERBOSE('SMB Kernel thread exiting (%d)...\n',
current->pid);
340 module_put_and_exit(0);
341 }
342
As is clear from the code, smbiod is a thread that runs in a continuous loop as it processes I/O requests. When there are no I/O requests to process, the thread goes to sleep on the wait queue smbiod_wait. This is achieved by calling wait_event_interruptible (line 304). This call causes the smbiod to sleep only if the DATA_READY bit is set. As mentioned earlier, wait_event_interruptible takes care to avoid the lost wake-up problem.
Now, when a process wants to get some I/O done, it sets the DATA_READY bit in the smbiod_flags and wakes up the smbiod thread to perform I/O. This can be seen in the following code snippet (linux-2.6.11/fs/smbfs/smbiod.c: 57):
57 void smbiod_wake_up(void)
58 {
59 if (smbiod_state == SMBIOD_DEAD)
60 return;
61 set_bit(SMBIOD_DATA_READY, &smbiod_flags);
62 wake_up_interruptible(&smbiod_wait);
63 }
wake_up_interruptible wakes up one process that was sleeping on the smbiod_wait waitqueue. The function smb_add_request (linux-2.6.11/fs/smbfs/request.c: 279) calls the smbiod_wake_up function when it adds new requests for processing.
Thundering Herd Problem
Another classical operating system problem arises due to the use of the wake_up_all function. Let us consider a scenario in which a set of processes are sleeping on a wait queue, wanting to acquire a lock.
Once the process that has acquired the lock is done with it, it releases the lock and wakes up all the processes sleeping on the wait queue. All the processes try to grab the lock. Eventually, only one of these acquires the lock and the rest go back to sleep.
This behavior is not good for performance. If we already know that only one process is going to resume while the rest of the processes go back to sleep again, why wake them up in the first place? It consumes valuable CPU cycles and incurs context-switching overheads. This problem is called the thundering herd problem. That is why using the wake_up_all function should be done carefully, only when you know that it is required. Otherwise, go ahead and use the wake_up function that wakes up only one process at a time.
So, when would the wake_up_all function be used? It is used in scenarios when processes want to take a shared lock on something. For example, processes waiting to read data on a page could all be woken up at the same moment.
Time-Bound Sleep
You frequently may want to delay the execution of your process for a given amount of time. It may be required to allow the hardware to catch up or to carry out an activity after specified time intervals, such as polling a device, flushing data to disk or retransmitting a network request. This can be achieved by the function schedule_timeout(timeout), a variant of schedule(). This function puts the process to sleep until timeout jiffies have elapsed. jiffies is a kernel variable that is incremented for every timer interrupt.
As with schedule(), the state of the process has to be changed to TASK_INTERRUPTIBLE/TASK_UNINTERRUPTIBLE before calling this function. If the process is woken up earlier than timeout jiffies have elapsed, the number of jiffies left is returned; otherwise, zero is returned.
Let us take a look at a real-life example (linux-2.6.11/arch/i386/kernel/apm.c: 1415):
1415 set_current_state(TASK_INTERRUPTIBLE);
1416 for (;;) {
1417 schedule_timeout(APM_CHECK_TIMEOUT);
1418 if (exit_kapmd)
1419 break;
1421 * Ok, check all events, check for idle
.... * (and mark us sleeping so as not to
.... * count towards the load average)..
1423 */
1424 set_current_state(TASK_INTERRUPTIBLE);
1425 apm_event_handler();
1426 }
This code belongs to the APM thread. The thread polls the APM BIOS for events at intervals of APM_CHECK_TIMEOUT jiffies. As can be seen from the code, the thread calls schedule_timeout() to sleep for the given duration of time, after which it calls apm_event_handler() to process any events.
You also may use a more convenient API, with which you can specify time in milliseconds and seconds:
1.
msleep(time_in_msec);
2.
msleep_interruptible(time_in_msec);
3.
ssleep(time_in_sec);
msleep(time_in_msec); and msleep_interruptible(time_in_msec); accept the time to sleep in milliseconds, while ssleep(time_in_sec); accepts the time to sleep in seconds. These higher-level routines internally convert the time into jiffies, appropriately change the state of the process and call schedule_timeout(), thus making the process sleep.
I hope that you now have a basic understanding of how processes safely can sleep and wake up in the kernel. To understand the internal working of wait queues and advanced uses, look at the implementations of init_waitqueue_head, as well as variants of wait_event and wake_up.
Acknowledgement
Greg Kroah-Hartman reviewed a draft of this article and contributed valuable suggestions.
Kedar Sovani (www.geocities.com/kedarsovani) works for Kernel Corporation as a kernel developer. His areas of interest include security, filesystems and distributed systems."
Thursday, December 10, 2009
Gap buffer - Wikipedia, the free encyclopedia
From Wikipedia, the free encyclopedia
Jump to: navigation, search
In computer science, a gap buffer is a dynamic array that allows efficient insertion and deletion operations clustered near the same location. Gap buffers are especially common in text editors, where most changes to the text occur at or near the current location of the cursor. The text is stored in a large buffer in two contiguous segments, with a gap between them for inserting new text. Moving the cursor involves copying text from one side of the gap to the other (sometimes copying is delayed until the next operation that changes the text). Insertion adds new text at the end of the first segment. Deletion increases the size of the gap.
The advantage of using a gap buffer over more sophisticated data structures (such as linked lists) is that the text is represented simply as two literal strings, which take very little extra space and which can be searched and displayed very quickly.
The disadvantage is that operations at different locations in the text and ones that fill the gap (requiring a new gap to be created) require re-copying most of the text, which is especially inefficient for large files. The use of gap buffers is based on the assumption that such recopying occurs rarely enough that its cost can be amortized over the more common cheap operations.
A gap buffer is used in most Emacs editors.
[edit] Example
Below are some examples of operations with buffer gaps. The gap is represented pictorially by the empty space between the square brackets. This representation is a bit misleading: in a typical implementation, the endpoints of the gap are tracked using pointers or array indices, and the contents of the gap are ignored; this allows, for example, deletions to be done by adjusting a pointer without changing the text in the buffer. It is a common programming practice to use a semi-open interval for the gap pointers, i.e. the start-of-gap points to the invalid character following the last character in the first buffer, and the end-of-gap points to the first valid character in the second buffer (or equivalently, the pointers are considered to point "between" characters).
Initial state:
This is the way [ ]out.
User inserts some new text:
This is the way the world started [ ]out.
User moves the cursor before "started"; system moves "started " from the first buffer to the second buffer.
This is the way the world [ ]started out.
User adds text filling the gap; system creates new gap:
This is the way the world as we know it [ ]started out.
[edit] See also
* Dynamic array, the special case of a gap buffer where the gap is always at the end
* Linked list
* Circular buffer
* Rope (computer science)
[edit] External references
* Overview and implementation in .NET/C#
* Brief overview and sample C++ code
* Implementation of a cyclic sorted gap buffer in .NET/C#
* Use of gap buffer in early editor. (First written somewhere between 1969 and 1971)
Sunday, December 6, 2009
mutex-design.txt
2
3started by Ingo Molnar
4
5 "Why on earth do we need a new mutex subsystem, and what's wrong
6 with semaphores?"
7
8firstly, there's nothing wrong with semaphores. But if the simpler
9mutex semantics are sufficient for your code, then there are a couple
10of advantages of mutexes:
11
12 - 'struct mutex' is smaller on most architectures: .e.g on x86,
13 'struct semaphore' is 20 bytes, 'struct mutex' is 16 bytes.
14 A smaller structure size means less RAM footprint, and better
15 CPU-cache utilization.
16
17 - tighter code. On x86 i get the following .text sizes when
18 switching all mutex-alike semaphores in the kernel to the mutex
19 subsystem:
20
21 text data bss dec hex filename
22 3280380 868188 396860 4545428 455b94 vmlinux-semaphore
23 3255329 865296 396732 4517357 44eded vmlinux-mutex
24
25 that's 25051 bytes of code saved, or a 0.76% win - off the hottest
26 codepaths of the kernel. (The .data savings are 2892 bytes, or 0.33%)
27 Smaller code means better icache footprint, which is one of the
28 major optimization goals in the Linux kernel currently.
29
30 - the mutex subsystem is slightly faster and has better scalability for
31 contended workloads. On an 8-way x86 system, running a mutex-based
32 kernel and testing creat+unlink+close (of separate, per-task files)
33 in /tmp with 16 parallel tasks, the average number of ops/sec is:
34
35 Semaphores: Mutexes:
36
37 $ ./test-mutex V 16 10 $ ./test-mutex V 16 10
38 8 CPUs, running 16 tasks. 8 CPUs, running 16 tasks.
39 checking VFS performance. checking VFS performance.
40 avg loops/sec: 34713 avg loops/sec: 84153
41 CPU utilization: 63% CPU utilization: 22%
42
43 i.e. in this workload, the mutex based kernel was 2.4 times faster
44 than the semaphore based kernel, _and_ it also had 2.8 times less CPU
45 utilization. (In terms of 'ops per CPU cycle', the semaphore kernel
46 performed 551 ops/sec per 1% of CPU time used, while the mutex kernel
47 performed 3825 ops/sec per 1% of CPU time used - it was 6.9 times
48 more efficient.)
49
50 the scalability difference is visible even on a 2-way P4 HT box:
51
52 Semaphores: Mutexes:
53
54 $ ./test-mutex V 16 10 $ ./test-mutex V 16 10
55 4 CPUs, running 16 tasks. 8 CPUs, running 16 tasks.
56 checking VFS performance. checking VFS performance.
57 avg loops/sec: 127659 avg loops/sec: 181082
58 CPU utilization: 100% CPU utilization: 34%
59
60 (the straight performance advantage of mutexes is 41%, the per-cycle
61 efficiency of mutexes is 4.1 times better.)
62
63 - there are no fastpath tradeoffs, the mutex fastpath is just as tight
64 as the semaphore fastpath. On x86, the locking fastpath is 2
65 instructions:
66
67 c0377ccb
68 c0377ccb: f0 ff 08 lock decl (%eax)
69 c0377cce: 78 0e js c0377cde <.text.lock.mutex>
70 c0377cd0: c3 ret
71
72 the unlocking fastpath is equally tight:
73
74 c0377cd1
75 c0377cd1: f0 ff 00 lock incl (%eax)
76 c0377cd4: 7e 0f jle c0377ce5 <.text.lock.mutex+0x7>
77 c0377cd6: c3 ret
78
79 - 'struct mutex' semantics are well-defined and are enforced if
80 CONFIG_DEBUG_MUTEXES is turned on. Semaphores on the other hand have
81 virtually no debugging code or instrumentation. The mutex subsystem
82 checks and enforces the following rules:
83
84 * - only one task can hold the mutex at a time
85 * - only the owner can unlock the mutex
86 * - multiple unlocks are not permitted
87 * - recursive locking is not permitted
88 * - a mutex object must be initialized via the API
89 * - a mutex object must not be initialized via memset or copying
90 * - task may not exit with mutex held
91 * - memory areas where held locks reside must not be freed
92 * - held mutexes must not be reinitialized
93 * - mutexes may not be used in hardware or software interrupt
94 * contexts such as tasklets and timers
95
96 furthermore, there are also convenience features in the debugging
97 code:
98
99 * - uses symbolic names of mutexes, whenever they are printed in debug output
100 * - point-of-acquire tracking, symbolic lookup of function names
101 * - list of all locks held in the system, printout of them
102 * - owner tracking
103 * - detects self-recursing locks and prints out all relevant info
104 * - detects multi-task circular deadlocks and prints out all affected
105 * locks and tasks (and only those tasks)
106
107Disadvantages
108-------------
109
110The stricter mutex API means you cannot use mutexes the same way you
111can use semaphores: e.g. they cannot be used from an interrupt context,
112nor can they be unlocked from a different context that which acquired
113it. [ I'm not aware of any other (e.g. performance) disadvantages from
114using mutexes at the moment, please let me know if you find any. ]
115
116Implementation of mutexes
117-------------------------
118
119'struct mutex' is the new mutex type, defined in include/linux/mutex.h
120and implemented in kernel/mutex.c. It is a counter-based mutex with a
121spinlock and a wait-list. The counter has 3 states: 1 for "unlocked",
1220 for "locked" and negative numbers (usually -1) for "locked, potential
123waiters queued".
124
125the APIs of 'struct mutex' have been streamlined:
126
127 DEFINE_MUTEX(name);
128
129 mutex_init(mutex);
130
131 void mutex_lock(struct mutex *lock);
132 int mutex_lock_interruptible(struct mutex *lock);
133 int mutex_trylock(struct mutex *lock);
134 void mutex_unlock(struct mutex *lock);
135 int mutex_is_locked(struct mutex *lock);
136 void mutex_lock_nested(struct mutex *lock, unsigned int subclass);
137 int mutex_lock_interruptible_nested(struct mutex *lock,
138 unsigned int subclass);
139
Saturday, December 5, 2009
C++ Random Numbers - C++
1. use srand(time(0)) as a seed for random number generator. Note that we only need to call srand just once even if we generate a lot of rand numbers.
2. we can use rand( ) % (r - l + 1) + l to generate numbers between [l,u] with equal probability. However, there is another method of using l + (rand( ) * (r - l + 1))/(RAND_MAX + 1.0) to get the numbers. I did not see any explanations why the later one is better.
C++ Random Numbers
This post is useful and well-written 0 This post is unclear
#1
Nov 16th, 2003
Intro
This tutorial provides a brief introduction to the random number functions that come as part of the C++ standard library, namely rand() and srand().
rand() and RAND_MAX
The C++ standard library includes a pseudo random number generator for generating random numbers. In order to use it we need to include the
Here's a piece of code that will generate a single random number:
Help with Code Tags
C++ Syntax (Toggle Plain Text)
1.
#include
2.
#include
3.
4.
using namespace std;
5.
6.
int main()
7.
{
8.
int random_integer = rand();
9.
cout << random_integer << endl;
10.
}
#include
The value of RAND_MAX varies between compilers and can be as low as 32767, which would give a range from 0 to 32767 for rand(). To find out the value of RAND_MAX for your compiler run the following small piece of code:
Help with Code Tags
C++ Syntax (Toggle Plain Text)
1.
#include
2.
#include
3.
4.
using namespace std;
5.
6.
int main()
7.
{
8.
cout << "The value of RAND_MAX is " << RAND_MAX << endl;
9.
}
#include
srand()
The pseudo random number generator produces a sequence of numbers that gives the appearance of being random, when in fact the sequence will eventually repeat and is predictable.
We can seed the generator with the srand() function. This will start the generator from a point in the sequence that is dependent on the value we pass as an argument. If we seed the generator once with a variable value, for instance the system time, before our first call of rand() we can generate numbers that are random enough for simple use (though not for serious statistical purposes).
In our earlier example the program would have generated the same number each time we ran it because the generator would have been seeded with the same default value each time. The following code will seed the generator with the system time then output a single random number, which should be different each time we run the program.
Help with Code Tags
C++ Syntax (Toggle Plain Text)
1.
#include
2.
#include
3.
#include
4.
5.
using namespace std;
6.
7.
int main()
8.
{
9.
srand((unsigned)time(0));
10.
int random_integer = rand();
11.
cout << random_integer << endl;
12.
}
#include
Don't make the mistake of calling srand() every time you generate a random number; we only usually need to call srand() once, prior to the first call to rand().
Generating a number in a specific range
If we want to produce numbers in a specific range, rather than between 0 and RAND_MAX, we can use the modulo operator. It's not the best way to generate a range but it's the simplest. If we use rand()%n we generate a number from 0 to n-1. By adding an offset to the result we can produce a range that is not zero based. The following code will produce 20 random numbers from 1 to 10:
Help with Code Tags
C++ Syntax (Toggle Plain Text)
1.
#include
2.
#include
3.
#include
4.
5.
using namespace std;
6.
7.
int main()
8.
{
9.
srand((unsigned)time(0));
10.
int random_integer;
11.
for(int index=0; index<20; index++){
12.
random_integer = (rand()%10)+1;
13.
cout << random_integer << endl;
14.
}
15.
}
#include
A better method, though slightly more complicated, is given below. This overcomes problems that are sometimes experienced with some types of pseudo random number generator that might be supplied with your compiler. As before, this will output 20 random numbers from 1 to 10.
Help with Code Tags
C++ Syntax (Toggle Plain Text)
1.
#include
2.
#include
3.
#include
4.
5.
using namespace std;
6.
7.
int main()
8.
{
9.
srand((unsigned)time(0));
10.
int random_integer;
11.
int lowest=1, highest=10;
12.
int range=(highest-lowest)+1;
13.
for(int index=0; index<20; index++){
14.
random_integer = lowest+int(range*rand()/(RAND_MAX + 1.0));
15.
cout << random_integer << endl;
16.
}
17.
}
#include
Conclusion
If you need to use a pseudo random number generator for anything even remotely serious you should avoid the simple generator that comes with your compiler and use something more sophisticated instead. That said, rand() still has its place and you may find it useful.
Wednesday, December 2, 2009
Ubuntu下安装LXR - 迷失自我 - 51CTO技术博客
1.安装apache2
sudo apt-get install apache2
2.安装lxr
sudo apt-get install lxr
3. 在/etc/apache2/httpd.conf 末尾加上以下内容:
Alias /lxr /usr/share/lxr
Options All
AllowOverride All
这样可以达到[url]http://localhost/lxr/[/url] =>/usr/share/lxr
4. 在/usr/share/lxr/http下创建文件 .htaccess, 并写入一下内容:
SetHandler cgi-.
5.
sudo /etc/init.d/apache2 restart
6. 创建/usr/share/lxr/source/XX目录 (XX为版本号)
mkdir /usr/share/lxr/source/2.6.22
然后在/usr/share/lxr/source/2.6.22 下创建linux符号连接
ln -s /usr/src/linux-source-2.6.22 /usr/share/lxr/source/2.6.22/linux
7. 创建/usr/share/lxr/source/versions,这里记录所有要看的版本,内容是
2.6.20
2.6.22
要保证2.6.22 =>/usr/share/lxr/source/2.6.22
创建/usr/share/lxr/source/defversion,这里记录缺省要看的版本,内容是
2.6.22
之所以是这两个文件,见/usr/share/lxr/http/lxr.conf里的相关设置
8. 建立索引
cd /usr/share/lxr/source/2.6.22/
sudo genxref 2.6.22 //这样会在当前目录生成fileidx和xref
sudo glimpseindex -H /usr/share/lxr/source/2.6.22/ /usr/share/lxr/source/2.6.22/linux
(需要等待一段时间)
之所以是这个目录(/usr/share/lxr/source/2.6.22/),见/usr/share/lxr/http/lxr.conf里的相关设置(database项)
8.修改属性
sudo chmod +r -R /usr/share/lxr/source/2.6.22/*
9.
sudo /etc/init.d/apache2 restart
[url]http://localhost/lxr/http/blurb.html[/url]
原文地址:[url]http://hi.baidu.com/fanzier/blog/item/3ad7d7546f58a55dd009066b.html[/url]
Tuesday, December 1, 2009
待字闺中版之FAQ - 未名空间(mitbbs.com)
标 题: 待字闺中版之FAQ
发信站: BBS 未名空间站 (Thu Oct 19 22:07:13 2006)
1.找工作时最常遇见的各类问题及解答(questions and answers for job hunting)
http://www.JiansNet.com/ 剑知小站, 北美中文实用信息
http://www.JiansNet.com/list?label=4 美国工作, 计算机专业面试算法题和解答
2. 简历。
2.1. 简历的resume objective要针对一个特定的行业/公司/职位有的放矢,
不要写一个很笼统的objective希望可以放之四海而皆准。
或者你也可以写个summary of qualifications/highlight来代替objective。
2.2. 简历的作用是取得面试。所以简历的关键是要用最简略的语言把你符合某一职位要
求的亮点表达出来。
2.3. 应该准备两种格式的简历,一份WORD,一份ASCII。
如果你把简历放在EMAIL主体里,寄给人家,就应该用ASCII格式的。
3.找工作。
3.1. 如果你把简历放在找工作网站比如MONSTER里,应该在标题和简历里多用一些和
你专业相关的关键字,这样方便猎头找到你的简历。有时间的话还应该经常UPDATE你的简历。
3.2. 除了大的工作网站比如MONSTER, CAREERBUILDER等以外,还可以查看一些专业
和地区性比较强的工作网站。许多专业协会的网站也会有就业专栏。具体网站可以参考http://www.job-employment-guide.com/job-search.html以及本版精华区。
3.3. allaboutstat收录的找工作/学校申请宝典
http://www.geocities.com/allaboutstat/
4. 面试
4.1. 和面试找工作有关的电子书
http://www.job-employment-guide.com/free-ebooks.html(密码mitbbs)
http://share.mitbbs.googlepages.com/home
4.2.更多书籍,主要是IT,EE类的
http://www.megaupload.com/?d=1PPBNMZA (provided by ppzz)
这个网站也可做参考:
http://www.careercup.com/
还有这个网页:《计算机科学经典著作》(Computer Science)
http://lib.verycd.com/2004/12/16/0000031024.html
http://www.greatall.com/pages/2007/1/12/greatall_bt_item792436.html
4.3.Background check查什么?
精华区 -〉目录:Reference,Background Check和其他
4.4 常见面试问题(both traditional and behavioral)
http://www.quintcareers.com/interview_question_database/interview_questions_database.html
5. 工资谈判
5.1. 工资谈判的关键一是要知道这个职位的市场价是多少,二是最好不要先透露自己
的底牌。网上查工资的网站以下归纳的比较多:
http://www.job-employment-guide.com/job-salaries.html
不过有些信息比较老了,如果你能有同学/同事/朋友的建议,那当然是最可靠的了。
5.2. 最重要的是你的工资最好不要低于普遍工资水平(prevailing wage),否则会影响到
你H1和绿卡的申请。普遍工资可以在以下网址查询:http://www.flcdatacenter.com/
5.3. 你可以在劳工部查到以往H1B的所有数据,包括H1B申请者的雇主及工资,这是一
个很好的工资参考标准,这个网址同时也可以查到某个公司是否sponsor H1B。
http://www.flcdatacenter.com/CaseH1B.aspx
5.4. 分地点\职业查询工资(Fresh PhD一般为二级或者三级):
http://www.flcdatacenter.com/OesWizardStart.aspx
5.5. 另外一个查询以往H-1B的工资数据的网站
http://www.mydanwei.com/
5.6. 分享和查询工资的网站
http://www.glassdoor.com/member/home.htm
6. H1B (以下FAQ收入JHQ法律问题-关于H1专栏)
6.1. H1B标准说法,包括什么情况占名额,什么情况不占名额
精华区 -〉身份问题 -〉H-1B -〉H-1B 介绍 -〉文章:“H1B Introduction” 和 “
关于H-1B名额等问题的一个官方连接”
6.2. H-1B的生效方式和OPT的关系
精华区 -〉身份问题 -〉H-1B -〉Mandman专辑
6.3. 谁能解释一下为什么5-8月份毕业不好
精华区 -〉毕业的注意事项
6.4. How do I obtain an INTERIM
精华区 -〉身份问题 -〉CPT/OPT -〉Interim OPT
6.5. H1B未生效的情况下, 能否换工作单位?
精华区 -〉身份问题 -〉H-1B -〉Mandman专辑
6.6. 2001年度到2007年度H-1B名额使用情况回顾,
精华区 -〉身份问题 -〉Mandman专辑 or 精华区 -〉H-1B
6.7. Cap-Exempted H-1B (不占名额的H-1B)
An institution of higher education and a related or affiliated nonprofit entity;
A non-profit research organization;
A governmental research organization
6.8. Prevailing Wage入门
精华区 -〉身份问题 -〉H-1B -〉H-1B 介绍 -〉Prevailing Wage
6.9. EB-1,EB-2, EB-2 NIW和EB-3的区别和联系
精华区 -〉身份问题 -〉Mandman专辑 or 精华区 -〉绿卡
6.10. 申请H1B要提交哪些资料?
精华区 -〉身份问题 -〉H-1B -〉H-1B DIY
6.11. 2006年H-1B ADV的使用历程
精华区 -〉身份问题 -〉Mandman专辑
6.12. ABD H1-B(2007)
精华区 -〉身份问题 -〉H-1B -〉ABD
6.13. H1B CAP:
精华区 -〉H-1B -〉H-1B 介绍 -〉文章:“关于H-1B名额等问题的一个官方连接”
The H -1B Visa Reform Act of 2004, which took effect on May 5, 2005, changed
the H -1B filing procedures for FY 2005 and for future fiscal years. The Act
also makes available 20,000 new H -1B visas for foreign workers with a
master or higher level degree from a U.S. academic institution.
6.14. 根据receipt no.查USCIS处理状态(包括H-1B和OPT)
https://egov.uscis.gov/cris/caseStatusSearchDisplay.do
6.15. OPT批准日期汇总
http://www.trackitt.com/usa-immigration-trackers/opt-tracker/
http://trackgc.com/
7. 关于加急处理('EXPEDITE REQUEST') (切勿滥用!切记切记!VSC的FAX已经被废)
National Customer Service Call Center: 1-800-375-5283;
VSC Fax number: (802) 527-4816 (已停用);
CSC Fax number: (949) 389-8070 or (949) 389-8689;
TSC Fax number: (214) 962-2632 or (214) 962-1450;
NSC Fax number: (402) 219-6344/6170/6171/6325.
8. 身份的GAP
精华区 -〉毕业的注意事项 -> 身份的GAP
9. ICC黑名单
http://www.desicrunch.com/Companies.aspx
10. 查未来雇主是否E-verify
http://www.smartbusinesspractices.com/pilot/
11. 移民局批件(I-797)丢失或者被扣之后补办(需要Case number)
I-824, Application for Action on an Approved Application or Petition
http://www.uscis.gov/files/form/I-824.pdf"
Sunday, November 29, 2009
find the subvector with max sum in a circular array
output: a subvector of the array that has max sum
We can think that n elements are put on a circle and we want to find the subvector from the circle that has max sum.
Solution:
1. take any element as the leader and break the circle into one dimensional array a[1:n]. use the classical method to get the maxsum subvector
2. we did not consider the case that the maxsum subvector in the circle passes a[1]. in the next step we will figure out the maxsum subvector that passes a[1], suppose it is a[n - i:j].
3. claim: a[j+1:n-i-1] is the minsum subvector of a[1:n]. because sum of a[j+1:n-i-1] + sum of a[j+1:n-i-1] is fixed, one takes max means the other gets min.
Application:
find an index that the subvector starting from him always has sum greater than or equal to 0.
There are n gas stations posit... | CareerCup: "Bloomberg LP » Brain Teasers
DPS Prog on May 17, 2009 | Question #134799 (Report Dup) | Edit | History
There are n gas stations positioned along a circular road. Each has a limited supply of gas. You can only drive clockwise around the road. You start with zero gas. Knowing how much gas you need to get from each gas station to the next and how much gas you can get at each station, design an algorithm to find the gas station you need to start at to get all the way around the circle.
More Questions from this Interview
Filed Under: Bloomberg LP » Brain Teasers » Software Engineer / Developer
LOler on May 18, 2009 |Edit | Edit
O(n^2) time is easy. Start at each and just simulate it out.
O(n) time is possible, but I won''t spoil it for you.
Reply to Comment
CyberPhoenix on May 19, 2009 |Edit | Edit
Check Cormen for this, Its in exercise section of some chapters.....
Reply to Comment
Cookie on May 20, 2009 |Edit | Edit
Here is what Cormen has to say about the above problem but i quite well did not understand the solution..
The optimal strategy is the obvious greedy one. Starting will a full tank of gas,
Professor Midas should go to the farthest gas station he can get to within n miles
of Newark. Fill up there. Then go to the farthest gas station he can get to within n
miles of where he Þlled up, and Þll up there, and so on.
Looked at another way, at each gas station, Professor Midas should check whether
he can make it to the next gas station without stopping at this one. If he can, skip
this one. If he cannot, then Þll up. Professor Midas doesn’t need to know how
much gas he has or how far the next station is to implement this approach, since at
each Þllup, he can determine which is the next station at which he’ll need to stop.
This problem has optimal substructure. Suppose there are m possible gas stations.
Consider an optimal solution with s stations and whose Þrst stop is at the kth gas
station. Then the rest of the optimal solution must be an optimal solution to the
subproblem of the remaining m − k stations. Otherwise, if there were a better
solution to the subproblem, i.e., one with fewer than s −1 stops, we could use it to
come up with a solution with fewer than s stops for the full problem, contradicting
our supposition of optimality.
This problem also has the greedy-choice property. Suppose there are k gas stations
beyond the start that are within n miles of the start. The greedy solution chooses
the kth station as its Þrst stop. No station beyond the kth works as a Þrst stop,
since Professor Midas runs out of gas Þrst. If a solution chooses a station j < k as
its Þrst stop, then Professor Midas could choose the kth station instead, having at
least as much gas when he leaves the kth station as if he’d chosen the j th station.
Therefore, he would get at least as far without Þlling up again if he had chosen the
kth station.
If there are m gas stations on the map, Midas needs to inspect each one just once.
The running time is O(m).
Reply to Comment
Marian on May 26, 2009 |Edit | Edit
Cookie, no, that's not the problem. Therefore that's not the solution :))
I won't give the full solution how to do it in O(n), but I will provide all the hints.
The problem can be cast into a modified version of the very know problem: Maximum Consecutive Subsequence (of length 2*n-1). If during the search (in linear time for Maximum Consecutive Subsequence) you find a positive sum of length n, you found the solution to this problem.
Reply to Comment
karthikvaidy on June 12, 2009 |Edit | Edit
For each station, find (distance that can be traveled with gas available in that station - distance to next station). Create an array of these values and find the maximum positive consecutive substring. You have to start driving at the beginning of this substring.
PS: Remember that the stations are circularly arranged, so should the array. So it is possible for you to start on the 'last' station and still go around to all of them.
PPS: Another thing that can be done is to ensure that sum of all distances to be traveled is less than distance that can be traveled with available gas. Otherwise, it makes not difference where you start, you will get stranded.
Reply to Comment
Bajanfella on June 14, 2009 |Edit | Edit
Basically you have two arrays, gas_needed[n] and gas_at_station[n]. However, I would work with a third array which is the difference gas_needed - gas_at_station. Let's call this array gas_remaining[n](R[n])
Sum(Ri) where m is the nth consecutive station after i. i is the starting station if
i->m
the sum never goes negative.
O(m)
Reply to Comment
noname on July 10, 2009 |Edit | Edit
Bajanfella, Your algorithm is O(m^2) instead of O(m). Basically, you are just simulating with different starting points.
Reply to Comment
smartAss on July 17, 2009 |Edit | Edit
making use of a part of data str given by BajanFella, Lets take all the three arrays. Now in the arrya gas_remaining[] find the maximum subsequence which can be done in O(m), now starting at the first element of the subsequence solves the problem, thats it, we're done...
Reply to Comment
googler on August 13, 2009 |Edit | Edit
bejafella's answer is the correct one...and I must say this quite a popular interview question!
Reply to Comment
john on August 19, 2009 |Edit | Edit
yes, bejafella's got it
Reply to Comment
vipul k. on September 21, 2009 |Edit | Edit
if i have a car with 'zero' gas, how would i start the car in the first place???
Reply to Comment
S.M on October 01, 2009 |Edit | Edit
What if the gas tank is limited in capacity ?"
Saturday, November 28, 2009
Fair division - Wikipedia, the free encyclopedia
From Wikipedia, the free encyclopedia
Fair division, also known as the cake cutting problem, is the problem of dividing a resource in such a way that all recipients believe that they have received a fair amount. The problem is easier when recipients have different measures of value of the parts of the resource: in the "cake cutting" version, one recipient may like marzipan, another prefers cherries, and so on—then, and only then, the n recipients may get even more than what would be one n-th of the value of the "cake" for each of them. On the other hand, the presence of different measures opens a vast potential (a Pandora box) for many challenging questions and directions of further research.
There are a number of variants of the problem. The definition of 'fair' may simply mean that they get at least their fair proportion, or harder requirements like envy-freeness may also need to be satisfied. The theoretical algorithms mainly deal with goods that can be divided without losing value. The division of indivisible goods, as in for instance a divorce, is a major practical problem. Chore division is a variant where the goods are undesirable.
Fair division is often used to refer to just the simplest variant. That version is referred to here as proportional division or simple fair division.
Most of what is normally called a fair division is not considered so by the theory because of the use of arbitration. This kind of situation happens quite often with mathematical theories named after real life problems. The decisions in the Talmud on entitlement when an estate is bankrupt reflect some quite complex ideas about fairness,[1] and most people would consider them fair. However they are the result of legal debates by rabbis rather than divisions according to the valuations of the claimants.
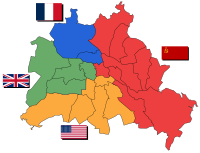
Contents[hide] |
[edit] Assumptions
Fair division is a mathematical theory based on an idealization of a real life problem. The real life problem is the one of dividing goods or resources fairly between people, the 'players', who have an entitlement to them. The central tenet of fair division is that such a division should be performed by the players themselves, maybe using a mediator but certainly not an arbiter as only the players really know how they value the goods.
The theory of fair division provides explicit criteria for various different types of fairness. Its aim is to provide procedures (algorithms) to achieve a fair division, or prove their impossibility, and study the properties of such divisions both in theory and in real life.
The assumptions about the valuation of the goods or resources are:
- Each player has their own opinion of the value of each part of the goods or resources
- The value to a player of any allocation is the sum of his valuations of each part. Often just requiring the valuations be weakly additive is enough.
- In the basic theory the goods can be divided into parts with arbitrarily small value.
Indivisible parts make the theory much more complex. An example of this would be where a car and a motorcycle have to be shared. This is also an example of where the values may not add up nicely, as either can be used as transport. The use of money can make such problems much easier.
The criteria of a fair division are stated in terms of a players valuations, their level of entitlement, and the results of a fair division procedure. The valuations of the other players are not involved in the criteria. Differing entitlements can normally be represented by having a different number of proxy players for each player but sometimes the criteria specify something different.
In the real world of course people sometimes have a very accurate idea of how the other players value the goods and they may care very much about it. The case where they have complete knowledge of each others valuations can be modeled by game theory. Partial knowledge is very hard to model. A major part of the practical side of fair division is the devising and study of procedures that work well despite such partial knowledge or small mistakes.
A fair division procedure lists actions to be performed by the players in terms of the visible data and their valuations. A valid procedure is one that guarantees a fair division for every player who acts rationally according to their valuation. Where an action depends on a player's valuation the procedure is describing the strategy a rational player will follow. A player may act as if a piece had a different value but must be consistent. For instance if a procedure says the first player cuts the cake in two equal parts then the second player chooses a piece, then the first player cannot claim that the second player got more.
What the players do is:
- Agree on their criteria for a fair division
- Select a valid procedure and follow its rules
It is assumed the aim of each player is to maximize the minimum amount they might get, or in other words, to achieve the maximin.
Procedures can be divided into finite and continuous procedures. A finite procedure would for instance only involve one person at a time cutting or marking a cake. Continuous procedures involve things like one player moving a knife and the other saying stop. Another type of continuous procedure involves a person assigning a value to every part of the cake.
[edit] Criteria for a fair division
There are a number of widely used criteria for a fair division. Some of these conflict with each other but often they can be combined. The criterion are described here only for when each player is entitled to the same amount.
- A proportional or simple fair division guarantees each player gets his fair share. For instance if three people divide up a cake each gets at least a third by their own valuation.
- An envy-free division guarantees no-one will want somebody else's share more than their own.
- An exact division is one where every player thinks everyone received exactly their fair share, no more and no less.
- An efficient or Pareto optimal division ensures no other allocation would make someone better off without making someone else worse off. The term efficiency comes from the economics idea of the efficient market. A division where one player gets everything is optimal by this definition so on its own this does not guarantee even a fair share.
- An equitable division is one where the proportion of the cake a player receives by their own valuation is the same for every player. This is a difficult aim as players need not be truthful if asked their valuation.
[edit] Two players
For two people there is a simple solution which is commonly employed. This is the so-called divide and choose method. One person divides the resource into what they believe are equal halves, and the other person chooses the "half" they prefer. Thus, the person making the division has an incentive to divide as fairly as possible: for if they do not, they will likely receive an undesirable portion. This solution satisfies gives a proportional and envy-free division.
The article on divide and choose describes why the procedure is not equitable. More complex procedures like the Adjusted Winner procedure are designed to cope with indivisible goods and to be more equitable in a practical context.
Austin's moving-knife procedure[2] gives an exact division for two players. The first player places two knives over the cake such that one knife is at the left side of the cake, and one is further right; half of the cake lies between the knives. He then moves the knives right, always ensuring there is half the cake – by his valuation – between the knives. If he reaches the right side of the cake, the leftmost knife must be where the rightmost knife started off. The second player stops the knives when he thinks there is half the cake between the knives. There will always be a point at which this happens, because of the intermediate value theorem.
The surplus procedure (SP) achieves a form of equitability called proportional equitability. This procedure is strategy proof and can be generalized to more than two people.[3]
[edit] Many players
Fair division with three or more players is considerably more complex than the two player case.
Proportional division is the easiest and the article describes some procedures which can be applied with any number of players. Finding the minimum number of cuts needed is an interesting mathematical problem.
Envy-free division was first solved for the 3 player case in 1960 independently by John Selfridge of Northern Illinois University and John Horton Conway at Cambridge University. The best algorithm uses at most 5 cuts.
The first cake cutting procedure for 4 or more players that produced an envy-free division of cake for any number of persons was first published by Steven Brams and Alan Taylor in 1992.[4] This number of cuts that might be required by this procedure is unbounded. A bounded moving knife procedure for 4 players was found in 1997.
There are no discrete algorithms for an exact division even for two players, a moving knife procedure is the best that can be done. There are no exact division algorithms for 3 or more players but there are 'near exact' algorithms which are also envy-free and can achieve any desired degree of accuracy.
A generalization of the surplus procedure called the equitable procedure (EP) achieves a form of equitability. Equitability and envy-freeness can be incompatible for 3 or more players.[3]
[edit] Variants
Some cake-cutting procedures are discrete, whereby players make cuts with a knife (usually in a sequence of steps). Moving-knife procedures, on the other hand, allow continuous movement and can let players call "stop" at any point.
A variant of the fair division problem is chore division: this is the "dual" to the cake cutting problem in which an undesirable object is to be distributed amongst the players. The canonical example is a set of chores that the players between them must do. Note that "I cut, you choose" works for chore division.
Sperner's Lemma can be used to get as close an approximation as desired to an envy-free solutions for many players. The algorithm gives a fast and practical way of solving some fair division problems.[5][6][7]
The division of property, as happens for example in divorce or inheritance, normally contains indivisible items which must be fairly distributed between players, possibly with cash adjustments (such pieces are referred to as atoms).
A common requirement for the division of land is that the pieces be connected, i.e. only whole pieces and not fragments are allowed. For example the division of Berlin after World War 2 resulted in four connected parts.
A consensus halving is where a number of people agree that a resource has been evenly split in two, this is described in exact division.
[edit] History
According to Sol Garfunkel, the cake cutting problem had been one of the most important open problems in 20th century mathematics,[8] when the most important variant of the problem was finally solved together by Steven Brams and Alan Taylor in 1995.
Divide and choose has probably been used since prehistory. The related activities of bargaining and barter are also ancient. Negotiations involving more than two people are also quite common, the Potsdam Conference is a notable recent example.
The theory of fair division dates back only to the end of the second world war. It was devised by a group of Polish mathematicians, Hugo Steinhaus, Bronisław Knaster and Stefan Banach, who used to meet in the Scottish Café in Lvov (then in Poland). A proportional (fair division) division for any number of players called 'last-diminisher' was devised in 1944. This was attributed to Banach and Knaster by Steinhaus when he made the problem public for the first time at a meeting of the Econometric Society in Washington D.C. on 17 September 1947. At that meeting he also proposed the problem of finding the smallest number of cuts necessary for such divisions.
Envy-free division was first solved for the 3 player case in 1960 independently by John Selfridge of Northern Illinois University and John Horton Conway at Cambridge University, the algorithm was first published in the 'Mathematical Games' column by Martin Gardner in Scientific American.
Envy-free division for 4 or more players was a difficult open problem of the twentieth century. The first cake cutting procedure that produced an envy-free division of cake for any number of persons was first published by Steven Brams and Alan Taylor in 1992.
A major advance on equitable division was made in 2006 by Steven J. Brams, Michael A. Jones, and Christian Klamler.[3]
[edit] In popular culture
In Numb3rs season 3 episode 'One Hour' Charlie talks about the cake-cutting problem as applied to the amount of money a kidnapper was demanding.
Hugo Steinhaus wrote about a number of variants of fair division in his book 'Mathematical Snapshots'. In his book he says a special 3 person version of fair division was devised by G. Krochmainy in Berdechów in 1944 and another by Mrs L Kott.[9]
Martin Gardner and Ian Stewart have both published books with sections about the problem.[10][11] Martin Gardner introduced the chore division form of the problem. Ian Stewart has popularized the fair division problem with his articles in Scientific American and New Scientist.
A Dinosaur comics strip is based on the cake-cutting problem.[12]
[edit] See also
[edit] References
- ^ Game Theoretic Analysis of a bankruptcy Problem from the Talmud Robert J. Aumann and Michael Maschler. Journal of Economic Theory 36, 195-213 (1985)
- ^ A.K. Austin. Sharing a Cake. Mathematical Gazette 66 1982
- ^ a b c Brams, Steven J.; Michael A. Jones and Christian Klamler (December 2006). "Better Ways to Cut a Cake" (PDF). Notices of the American Mathematical Society 53 (11): pp.1314–1321. http://www.ams.org/notices/200611/fea-brams.pdf. Retrieved 2008-01-16.
- ^ Steven J. Brams; Alan D. Taylor (January 1995). "An Envy-Free Cake Division Protocol". The American Mathematical Monthly (Mathematical Association of America) 102 (1): 9–18. doi:10.2307/2974850. http://www.jstor.org/pss/2974850. Retrieved 2008-11-15.
- ^ Rental Harmony: Sperner's Lemma in Fair Division Francis Edward Su. Amer. Math. Monthly, 106(1999), 930-942. (based on work by Forest Simmons 1980)
- ^ The Fair Division Calculator
- ^ http://www.maa.org/mathland/mathtrek_3_13_00.html A Fair Deal for Housemates. Ivars Peterson's MathTrek. March 13, 2000
- ^ Sol Garfunkel. More Equal than Others: Weighted Voting. For All Practical Purposes. COMAP. 1988
- ^ Mathematical Snapshots. H.Steinhaus. 1950, 1969 ISBN 0-19-503267-5
- ^ aha! Insight. Martin. Gardner, 1978. ISBN ISBN 978-0716710172
- ^ How to cut a cake and other mathematical conundrums. Ian Stewart. 2006. ISBN 978-0199205905
- ^ http://www.qwantz.com/archive/001345.html
[edit] Further reading
- Steven J. Brams and Alan D. Taylor (1996). Fair Division - From cake-cutting to dispute resolution Cambridge University Press. ISBN 0-521-55390-3
- Jack Robertson and William Webb (1998). Cake-Cutting Algorithms: Be Fair If You Can, AK Peters Ltd, . ISBN 1-56881-076-8.
[edit] External links
- Short essay about the cake-cutting problem by S. Abbas Raza of 3 Quarks Daily.
- Fair Division from the Discrete Mathematics Project at the University of Colorado at Boulder.
- The Fair Division Calculator (Java applet) at Harvey Mudd College
- Fair Division: Method of Lone Divider
- Fair Division: Method of Markers
- Fair Division: Method of Sealed Bids
- Vincent P. Crawford (1987). "fair division," The New Palgrave: A Dictionary of Economics, v. 2, pp. 274-75.
- Hal Varian (1987). "fairness," The New Palgrave: A Dictionary of Economics, v. 2, pp. 275-76.
- Bryan Skyrms (1996). The Evolution of the Social Contract Cambridge University Press. ISBN 9780521555838